Obtaining actionable solubility predictions with deepmirror
The ability to accurately predict molecular properties such as aqueous solubility can accelerate drug discovery by helping chemists to design the next round of compounds in the Design-Make-Test-Analyze (DMTA) cycle for a drug discovery program. Aqueous solubility in particular plays a vital role in determining a compound's bioavailability and overall drug-likeness. To predict solubility scientists frequently gather experimental data from lab assays to build QSAR models that are able to predict properties. In practice, these models are rarely accurate enough to be useful, either because there is not enough relevant data available, or because the molecules and assays used to train these models come from different projects that do not resemble the data that the models are applied to. The Sanders Tri-Institutional Therapeutics Discovery Institute (TDI), a non-profit drug discovery institute affiliated with Weill Cornell Medicine, The Rockefeller University, and Memorial Sloan Kettering Cancer Center, approached deepmirror with this problem. Even though the institute had access to large quantities of public and private data, they struggled to harness their data when working on new drug discovery programs and were unable to generate predictions that would be sufficiently accurate to become helpful and actionable. To help, deepmirror intelligently combined all of TDI’s solubility data collected with a consistent kinetic solubility assay over a decade from a range of different projects to finetune the deepmirror engine.
We evaluated performance on an isolated test set provided by TDI containing 342 solubility measurements on chemically distinct molecules from the historical data that were measured with a different assay provider.
Finetuning the deepmirror engine increased the R2 score from 0.2 to 0.6 on test molecules, demonstrating a significantly improved predictive performance, previously not achievable with other AI/ML approaches.
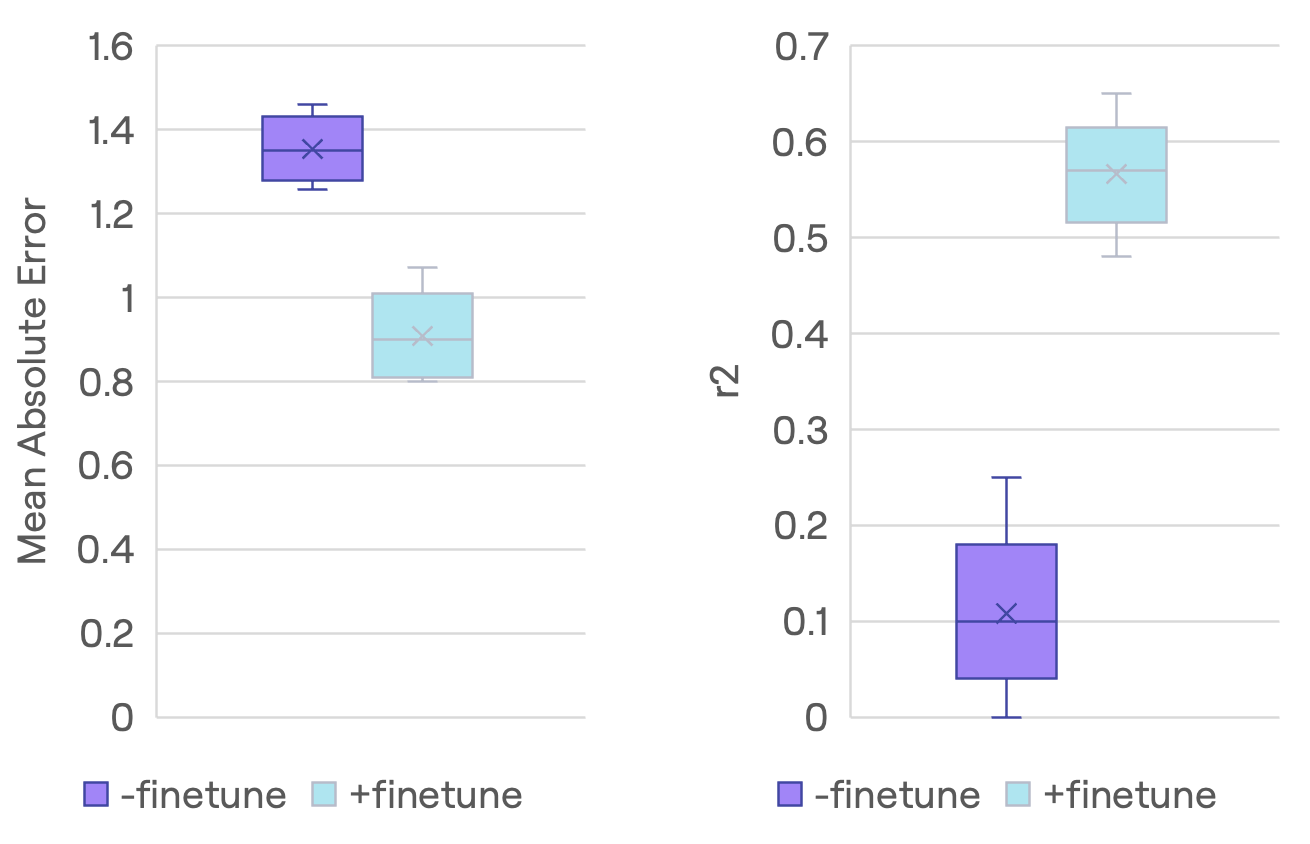
Figure: Direct comparison between Current App and FM model performance. The FM model achieves approximately 25% reduction in Mean Absolute Error (MAE) (from ~1.35 to ~0.95 logS) and a substantial improvement in R² score (from ~0.15 to ~0.58). Box plots show the statistical distribution with quartiles and whiskers representing the standard deviation. First, we randomly sampled 10% of the test data, corresponding to 38 molecules, for training and finetuning purposes and used the remaining 342 molecules as our test set. To ensure robust results, we repeated this sampling process 10 times and evaluated performance on the test data with the deepmirror engine with and without prior finetuning on historical TDI data. When sampling 30 molecules at random the fine-tuned engine improved the r2 from 0.15 to 0.58 while the mean absolute error decreased from ~1.35 to ~0.95. Note that models r2<0.5 are not very useful in practice.
This work demonstrates that finetuning of deepmirror’s predictive engine with historical data offers robust predictions with minimal training data, a typical situation when working on new programs.
This improved accuracy for novel chemical matter on novel programs significantly reduced dependence on large experimental datasets, and will enable supporting day to day drug design decisions for the wider chemistry team.
Acknowledgements
We thank our collaborators at the Sanders Tri-Institutional Therapeutics Discovery Institute for providing valuable data and feedback throughout this evaluation process.