The Path to AI-driven Drug Discovery - Part 2: How is AI disrupting Drug Discovery?
In our last blogpost, we introduced the classic drug-discovery pipeline to set the stage in which the AI-driven revolution is taking place. In Part 2, we will take a deep dive into the potential of AI in drug discovery.
Drug discovery is the process by which a new molecule is identified and optimised to treat patients. It is a multi-step and complex optimisation process with multiple cycles of drug selection, synthesis, and testing (Fig. 1). The main challenges are 1) the time and cost required to develop drugs and 2) the low success rates.
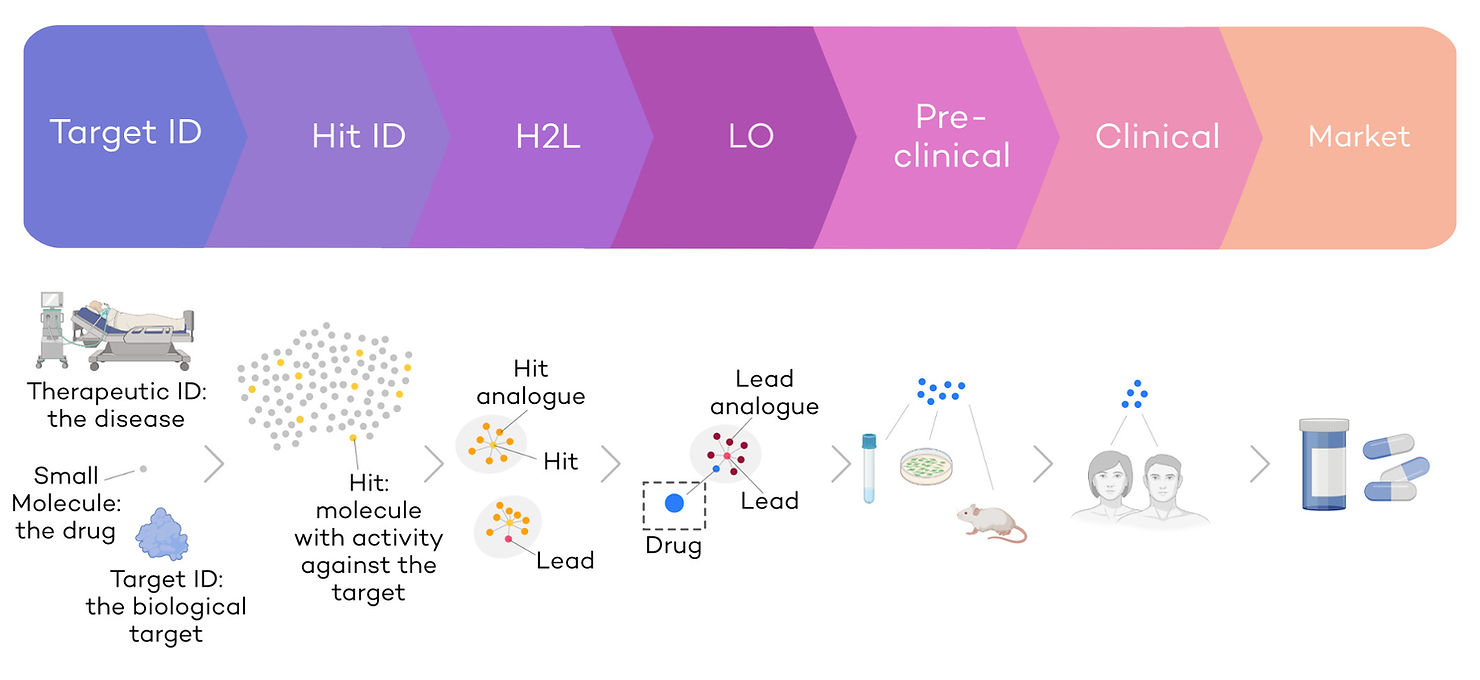
The development of a new small molecule drug requires 10-15 years and more than 1bn€ on average before it reaches the shelves (Wouters et al., 2020). On average, 3-5 years are invested in lead discovery and optimisation, and 9 years into clinical development (Brown et al., 2022; Eureka, Oct 21). Small molecule drug discovery is significantly slower that other discovery modalities such as therapeutic antibodies, where, on average, an antibody candidate takes 10-12 months from lead identification to phase 1 clinical trials (Jayatunga et al., 2022; Kelley, 2020). Despite the immense amount of effort and capital invested, small molecule drug success rates remain low: over 90% of drugs do not make it from Phase I clinical trials to approval (Mullard, 2016).
AI is poised to lower these barriers, as it has the potential to reduce time and cost, increase chances of clinical success (Jayatunga et al., 2022, Nature biopharma dealmakers News, May 2021).
How AI fast-tracks discovery
In 2022, a partnership between Evotec and Exscientia yielded a new anticancer drug candidate currently in Phase 1 clinical trials. Evotec is a large German biotechnology company with extensive expertise and capacity in large-scale drug discovery and marketing. Exscientia, on the other hand, is a relatively young AI-native drug discovery company, that spun-off the University of Dundee in 2012. Their collaboration identified an A2a receptor antagonist, a small molecule that can activate anti-tumour T cell activity and therefore promote removal of tumour cells. Instead of the conventional 4-5 years, Excientia/Evotech’s drug was identified in 8 months. Exscientia’s platform can generate novel compounds and predict their properties to rapidly evolve compounds until they have the desired predicted properties for clinical development. This analysis aids in prioritising compounds for synthesis. In classical drug discovery, candidates are selected based on much smaller amounts of information and require multiple iterative cycles of selection, synthesis, testing, and optimisation with many dead ends. Using AI, Exscientia fast-tracked candidate selection by ~4x (Nature biopharma dealmakers News, May 2021).
Companies like Exscientia are not alone. At least 10 other AI platform companies have established collaborations with pharmaceutical companies (Nature biopharma dealmakers News, May 2021) and are developing their own in-house testing capabilities for drug discovery – at least 24 of these companies have assets in pre-clinical and clinical development. Together, these companies have a combined discovery and pre-clinical output of 50% that of the top 20 ‘big pharma’, an astonishing proportion given AI for drug discovery is still a nascent industry (Jayatunga et al., 2022). The clinical success of these AI-driven discovery programmes is still to be seen, but given the large pre-clinical output, it is looking promising.
Where does DeepMirror fit into this?
AI-enabled drug discovery programmes often start by collaborations or partnerships between companies in which one side provides the AI and the other side provides laboratory and clinical capabilities (Fig. 2a). However, this approach requires recurring crosstalk between both companies, slowing down the process and reducing independence. Thus, companies tend to prefer developing internal AI capabilities (Fig. 2b). For example, pharmaceutical companies may build their own internal AI teams, or an AI-native company may develop drug testing capabilities to advance their own pipeline. However, building a new division within a company is a time-, labour- and capital-intensive approach. Even when a team is in place, it takes time and multiple iterations to develop an internal tool for laboratories to use.

These are the issues that DeepMirror is solving (Fig. 2c). We enable full control of AI-driven research from day one of a project. We provide our platform, DeepMirror Chem, as a no-code and intuitive software for R&D teams to carry out predictions (Fig. 3b). No need to engage with external stakeholders, build internal teams, or wait for tool development.
Our platform can be used across the drug discovery pipeline, for example in Hit-to-Lead to Lead Optimisation, with the ability to predict relevant properties such as target affinity, lipophilicity (logP), toxicity, ADME (Absorption, Distribution, Metabolism, and Excretion) or solubility, both from user data and our curated databases (Fig. 3a). Further laboratory results can be used to learn and refine predictions to generate insights to suggest the best drug candidates for further experimentation, ultimately facilitating the work of laboratories.
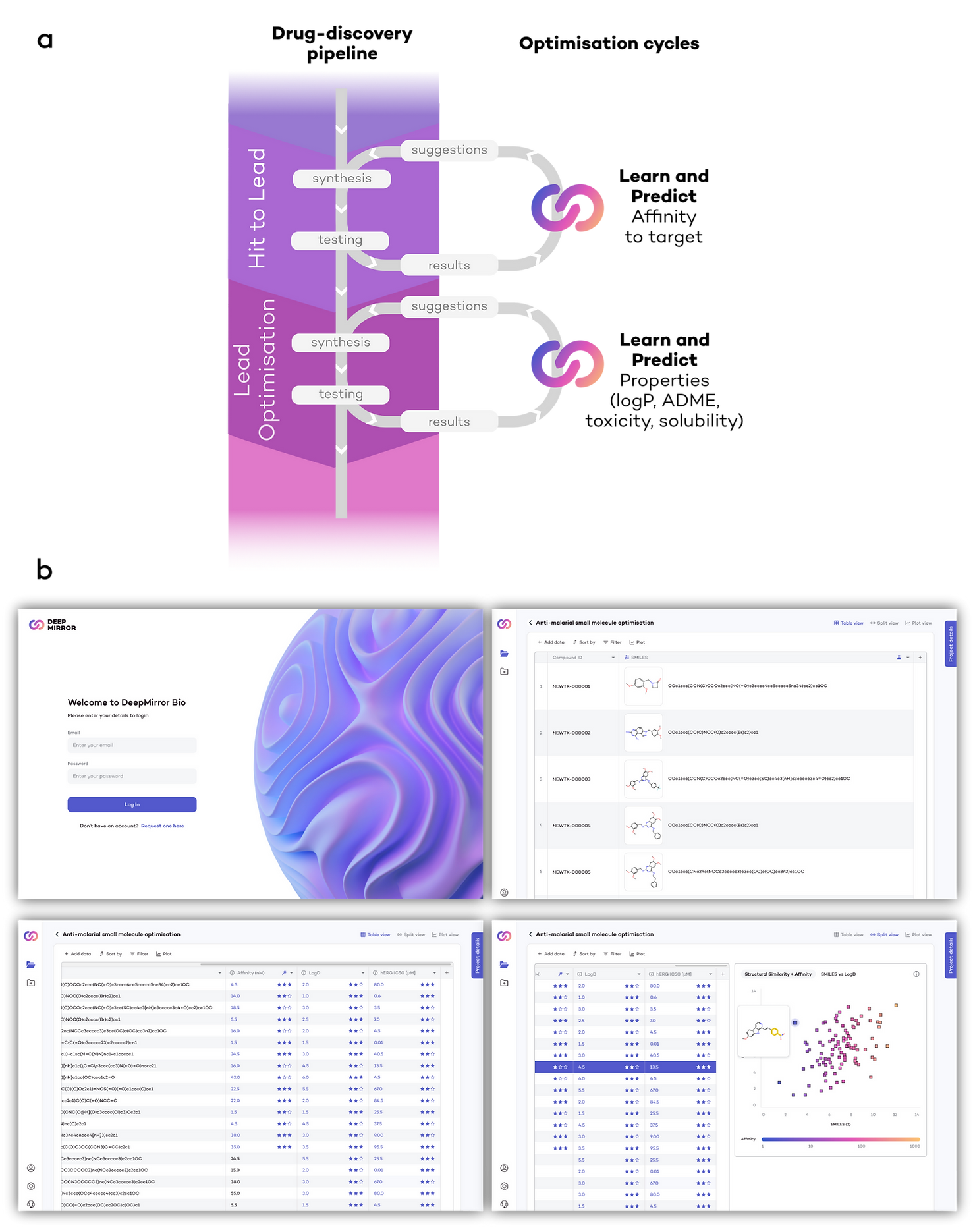
In the next post, we’ll bring a case study on how our platform fast-tracks drug discovery cycles and de-risks candidate selection. In the meantime, feel free to get in touch to book a demo of DeepMirror Bio!
References
Brown, D.G., Wobst, H.J., Kapoor, A., Kenna, L.A., Southall, N., 2022. Clinical development times for innovative drugs. Nat Rev Drug Discov 21, 793–794. https://doi.org/10.1038/D41573-021-00190-9
Eureka, Oct 21, Why Does Drug Development Take So Long? https://www.criver.com/eureka/why-does-drug-development-take-so-long
Jayatunga, M.K.P., Xie, W., Ruder, L., Schulze, U., Meier, C., 2022. AI in small-molecule drug discovery: a coming wave? Nat Rev Drug Discov 21, 175–176. https://doi.org/10.1038/D41573-022-00025-1
Kelley, B., 2020. Developing therapeutic monoclonal antibodies at pandemic pace. Nature Biotechnology 2020 38:5 38, 540–545. https://doi.org/10.1038/s41587-020-0512-5
Mullard, A., 2016. Parsing clinical success rates. Nat Rev Drug Discov 15, 447. https://doi.org/10.1038/NRD.2016.136
Nature biopharma dealmakers News, May 2021: https://www.nature.com/articles/d43747-021-00045-7
The Observer, Science, 18 Dec 2022: https://www.theguardian.com/science/2022/dec/18/the-10-biggest-science-stories-of-2022-chosen-by-scientists
Wouters, O.J., McKee, M., Luyten, J., 2020. Estimated Research and Development Investment Needed to Bring a New Medicine to Market, 2009-2018. JAMA 323, 844–853. https://doi.org/10.1001/JAMA.2020.116