The Path to AI-driven Drug Discovery - Part 3: AI to learn from lab data
In this blogpost series, we have so far outlined the process of drug discovery (Part 1) and taken a deep dive into the potential of AI-driven drug discovery (Part 2). In this third part, we show how AI tools, such as DeepMirror Chem, can fast-track affinity optimisation during hit-to-lead and lead optimisation in two real world cases. For more details, contact us to request our white paper!
AI to fast-track affinity optimisation in drug discovery
AI has shown great promise in fast-tracking drug discovery (Part 2). In the final blogpost of this series, we wanted to further explore with real data how AI can accelerate drug discovery.
As we’ve previously seen in Part 1 of the series, the first steps in drug discovery are i) identifying a biological target (related to a disease) (Target ID) and ii) finding a molecule that can bind with high affinity to it (Hit ID and H2L). We focused our attention on two targets: the main protease in SARS-CoV2, the virus that causes COVID-19, and HIF2a, a protein involved in cancer (Fig. 1). To identify which molecules can bind to these targets, a drug discovery researcher needs 1) to design an experiment selecting which molecules to test, and 2) test them in the lab to measure their affinity against a target. We called this an ‘experimental cycle’, in other words, the steps involved in each ‘batch’ of experiments (Fig 1).
Testing for affinity is carried out by measuring IC50, or the concentration required to reduce a target’s activity by half. The lower the IC50, the lower the amount of drug required to exert an effect on the target, therefore the higher the affinity. In other words, lower IC50 means higher affinity.

The COVID Moonshot and HIF2a inhibitors datasets
To simulate experimental cycles, we identified small molecules we could simulate testing. We curated two datasets containing lists of small molecules and their experimentally measured affinity (IC50) against the main protease in SARS-CoV2 and HIF2a. These datasets were the COVID Moonshot project dataset, and the results from a HIF2a inhibitor patent.
The COVID Moonshot project is an open-science, collaborative project spanning 150 scientists. It was born from a ‘twitter storm’ with the aim of quickly developing an anti-viral drug against COVID-19 (DNDi, COVID Moonshot). The dataset contains 2,062 molecules and their lab measured affinity (IC50). One of these molecules has successfully progressed into pre-clinical development. However, we didn’t know the identity of this molecule, so we defined ‘successful drug candidates’ as molecules with very high affinity with an IC50 < 0.05uM; 10 molecules in total.
The second dataset is a patent containing a collection of inhibitors against HIF2a and their lab measured IC50 (patent US-9908845-B2). The dataset contains 326 molecules of which 2 were selected for pre-clinical development because they had an IC50 < 0.01uM and good drug-like properties (which we will explore in a future case study).
Conventional vs AI-assisted drug discovery
To assess the effect of AI on the speed of drug discovery, we compared a simulated scenario where researchers did not use AI to design experiments (Conventional) (Fig 2, Scenario A), to a second simulation where they used AI to aid in designing experiments based on previous lab results (AI-assisted, powered by DeepMirror Chem) (Fig 2, Scenario B). To simulate testing of small molecules in the lab, we started with a dataset containing only the small molecules. Each time a molecule was selected for testing, we labelled its experimentally measured IC50 in the dataset. In both cases, we calculated the number of experimental cycles required to find (select for testing) real drug candidates.
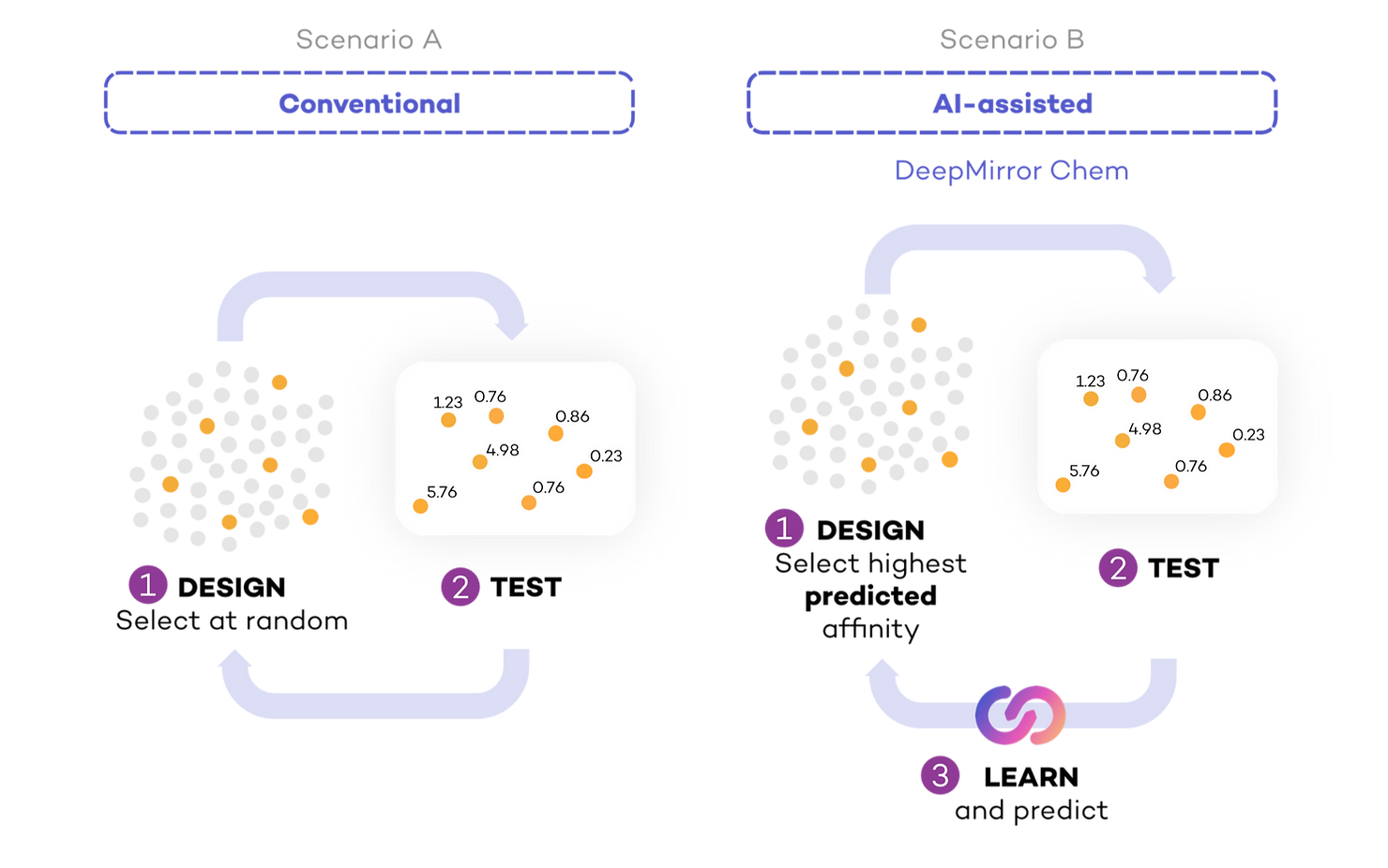
In conventional drug discovery, researchers decide on a shortlist of molecules to synthesise and test. We approximated conventional drug discovery by selecting sets of molecules at random for each cycle (30 for HIF2a inhibitors and 50 for COVID Moonshot) and subsequently testing these molecules. This step could be more complex, as research teams could include an expert medicinal chemist, who could help the small molecule selection with the intuition they built over many years.
In AI-assisted drug discovery, the same experimental cycle takes place, but researchers use the results of each cycle to systematically learn and inform the design of the next. To simulate AI-assisted drug discovery, we used DeepMirror Chem to suggest the first set of molecules to test. We used the results of these tests to predict the IC50 of the remaining untested molecules and selected the molecules with highest predicted affinity for the next round of testing. We kept repeating this process: selecting the highest predicted affinity molecules, testing them, and providing this new information to our platform. With every round, the algorithm had more information with which to better predict IC50.
For both datasets and both approaches (AI-assisted and Conventional), we stopped this iterative process once we found at least 5 (COVID Moonshot) or at least 2 (HIF2a inhibitors) high affinity (low IC50) molecules in the datasets.
2-4x acceleration of drug discovery
In our simulations, researchers assisted by AI (using DeepMirror Chem) could identify high affinity molecules 2-4 times quicker when compared to conventional drug discovery (Fig 3). At least 5 high affinity molecules against the main protease in SARS-Cov2 were found within 5 experimental cycles, while it took an average of 20 cycles for conventional drug discovery simulations (Fig 3a).

Similarly, DeepMirror Chem could find both HIF2a inhibitor candidates 2 times quicker than conventional drug discovery simulations (Fig 3b). On average, it took DeepMirror Chem 5 cycles to identify both pre-clinical candidates, versus an average of 11 cycles for conventional drug discovery simulations.
Take home message
The application of AI in drug discovery is already showing immense promise in reducing the discovery times of novel candidates. However, life sciences companies currently rely on i) creating partnerships with AI companies, which requires extensive management and communication, or ii) building internal teams and platforms, which is expensive and time-consuming (Part 2). In our previous blogpost, we identified what sets DeepMirror apart from AI consultancies or partnerships: we provide access to AI-driven drug discovery from day one via no-code and intuitive software. No need to engage with external stakeholders, build internal teams, or wait for tool development.
Hit-to-Lead, the stage at which affinity is most improved during drug discovery (See Part 1) requires on average 1.5 years and $2.5M, and Lead Optimisation requires 2 years and $10M (Paul et al., 2010). Together, that’s on average 3.5 years and $12.5M. AI has the potential to halve (or even reduce to a quarter!) the amount of time and cost invested in these drug discovery efforts. In this final blogpost of the series, we demonstrate, with real-world datasets, the increase in speed that AI enables compared to a conventional approach by using DeepMirror Chem to optimise small molecule affinity. DeepMirror Chem allows researchers to reap the benefits of adding AI into a pipeline without the worries of managing a partnership, building a team, or waiting for tool development, at a fraction of the cost.
If you want more technical detail about the inner workings of this case study or want to learn more about DeepMirror Chem, get in touch!
What about other properties?
Do you want to predict properties other than affinity, but do not have the experimental data yet? Using DeepMirror Chem, users can access certified models trained on our proprietary databases. Certified models can be used to predict small molecule properties such as solubility (lipophilicity), toxicity or metabolism (ADME) without any experimental data!
References
DNDi, COVID Moonshot Project https://dndi.org/research-development/portfolio/covid-moonshot/
Hif2a dataset, patent US-9908845-B2 https://pubchem.ncbi.nlm.nih.gov/patent/US-9908845-B2
Paul, S.M., Mytelka, D.S., Dunwiddie, C.T., Persinger, C.C., Munos, B.H., Lindborg, S.R., Schacht, A.L., 2010. How to improve R&D productivity: the pharmaceutical industry’s grand challenge. Nature Reviews Drug Discovery 2010 9:3 9, 203–214. https://doi.org/10.1038/nrd3078